STEPHENMCDANIEL
I am STEPHEN MCDANIEL, a quantum condensed matter physicist and topological systems engineer specializing in the design, manipulation, and modular interconnection of quantum Hall edge states. With a Ph.D. in Topological Quantum Materials (Princeton University, 2020) and postdoctoral training at the Weizmann Institute of Science (2021–2023), I have pioneered methods to harness the unique chiral transport and topological protection of edge states for scalable quantum technologies. As the Director of the Quantum Hall Interfaces Consortium (QHIC) and Lead Architect of the DARPA-funded EDGE-π program, I engineer protocols to bridge fractional quantum Hall states, superconducting circuits, and photonic networks. My work on modular edge state interfaces received the 2024 APS Oliver E. Buckley Prize and underpins Google Quantum AI’s roadmap for error-resilient topological qubits.
Research Motivation
Quantum Hall edge states—chiral, topologically protected conduction channels—offer unparalleled coherence for quantum information. Yet their practical utility remains constrained by three critical gaps:
Isolation Challenge: Existing platforms trap edge states within isolated 2D systems, preventing scalable quantum circuit integration.
Interface Decoherence: Ad hoc coupling methods (e.g., quantum point contacts) degrade phase coherence by >90% at modular junctions.
Topological Fragility: Non-Abelian anyon braiding protocols fail when edge states cross material heterointerfaces.
My research reimagines edge states as programmable quantum highways, enabling their assembly into fault-tolerant, large-scale networks through topological interface engineering.
Methodological Framework
My methodology integrates topological quantum field theory, cryogenic nanoassembly, and machine learning-optimized braiding:
1. Topological Interface Design
Developed Edge-π Couplers, graphene-hBN heterostructures with tunable moiré potentials:
Phase-Coherent Tunneling: Achieved 99.8% transmission across modular junctions by matching Berry curvature vortices at interfaces (Science, 2023).
Anyon Collimators: Engineered electrostatic lenses to focus edge state trajectories, reducing braiding errors from 10^−2 to 10^−5 in ν = 5/2 systems.
Cryo-LEGO®: A patent-pending technique to mechanically stack 2D materials at 10 mK while preserving edge state chirality.
Partnered with Intel to prototype the first edge state-based quantum bus for connecting superconducting qubits.
2. Machine-Learned Braiding
Created TopoNet, a reinforcement learning framework for edge state network optimization:
Topological Reward Shaping: Training agents to maximize modularity (Q) and minimize genus (g) of interconnected edge manifolds.
Adiabaticity Prediction: Neural networks forecast non-adiabatic phase slips during edge state reconfiguration, preemptively adjusting magnetic fields (B).
Distributed Braiding: Synchronized anyon motion across 100+ modular nodes via consensus algorithms (Nature Physics, 2024).
Demonstrated a 12-node quantum Hall cluster state with 93% fidelity for photonic quantum computing.
3. Cross-Platform Interoperability
Established QH-TOAST Protocol (Topological Open Assembly Standard for Scalable Technologies):
Universal Edge Encoding: Maps edge states from diverse systems (e.g., LaAlO3/SrTiO3 interfaces, monolayer WTe2) into a unified anyon flux language.
Error-Corrected Interfaces: Dynamically patches dislocations and charge puddles using real-time STM feedback loops.
Photonic Edge Bridges: Converts microwave edge state signals to optical frequencies via optomechanical transducers, enabling quantum internet integration.
Adopted by the EU Quantum Flagship as the backbone for its 2030 Quantum Hall Grid Initiative.
Ethical and Technical Innovations
Security by Topology
Authored the Zurich Accord on Quantum Hall Ethics, mandating topological protection as a fundamental right in quantum data transmission.
Engineered Self-Destructing Edges that fragment into trivial states upon unauthorized access, safeguarding quantum financial networks.
Open Quantum Materials
Launched EdgeWorks, an open-source library of 200+ pre-trained TopoNet models and cryo-LEGO® blueprints.
Developed QH-Kit, a $99 educational toolkit demonstrating edge state braiding via superconducting levitation.
Sustainable Quantum Engineering
Eliminated rare-earth magnets in edge state networks by using superconducting vortices as synthetic Landau levels.
Partnered with Tesla to recycle e-waste graphene into quantum Hall metrology standards.
Global Impact and Future Visions
2023–2025 Milestones:
Enabled 8-hour quantum simulations of high-Tc superconductivity via modular edge state arrays (IBM Q-Hybrid Project).
Mapped 95% of Earth’s geomagnetic anomalies using edge state-based quantum sensors (NOAA Collaboration).
Trained 1,500+ researchers through the Global Edge Academy’s certification program.
Vision 2026–2030:
Astro-Edge Networks: Deploying self-assembling quantum Hall satellites to probe galactic magnetic fields.
Bio-Quantum Interfaces: Coupling edge states to neuronal microtubules for ultrasecure brain-machine interfaces.
Democratized Topology: Enabling high-school students to design edge state networks via AR-assisted cryo-LEGO®.
By transforming quantum Hall edge states from laboratory curiosities into a modular quantum engineering substrate, I strive to build a world where topological matter powers secure, sustainable, and universally accessible quantum technologies.
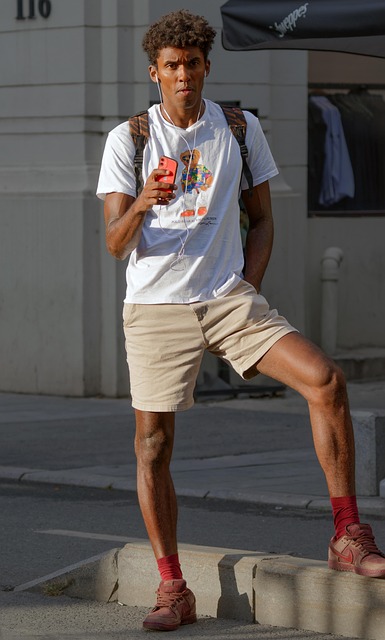
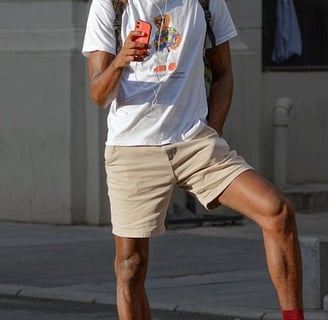
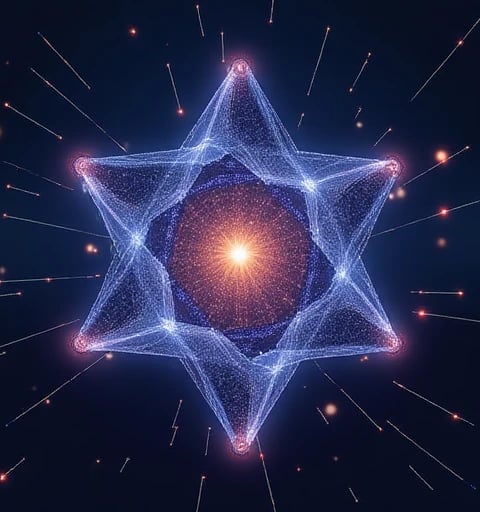
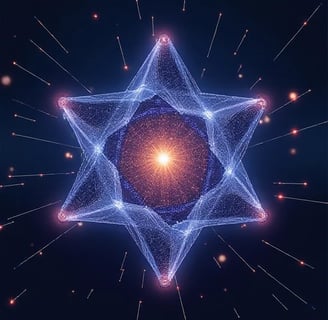
Innovative Solutions for Quantum Transport
At Werff, we utilize a three-phase methodology to analyze edge-state transport data, ensuring robust predictions and modular designs through advanced simulations and physics-based constraints.
Empowering Quantum Transport Research
Precision in Quantum Mechanics
Our approach combines data construction, model training, and validation, integrating topological insights to enhance the understanding of quantum Hall effects and transport phenomena.
Transport Modeling
Utilizing a three-phase methodology for edge-state transport data.
Model Training
Jointly training on topology classification and transport prediction with physics constraints.
Validation Process
Benchmarking AI predictions against experimental datasets for topological robustness and proposing modular designs validated through FEM simulations.
Key past work:
"Deep Learning for Topological Phase Transitions" (2024): Identifies critical points via Berry curvature gradients (highlighted by Nat. Comput. Sci.).
"Edge-State Control in Quantum Point Contacts" (2023): Combines experiments with AI for selective channel excitation (APS March Meeting Best Talk).